Abstract
Poplars (Populus alba, Populus canadensis, Populus canescens, Populus deltoides, Populus fremontii, Populus nigra and Populus simonii) are found throughout the world and are invasive in South Africa, where they are spatially permitted in certain areas under controlled conditions, as specified in the country’s invasive species legislation. To better trace their geographic distribution, this study predicts the potentially suitable habitat of poplar trees in South Africa based on generalised linear model (GLM), Random Forests (RF) and Support Vector Machines (SVM) models and also assesses the climatic variables with the greatest impact on prediction performance. The results show excellent performance for all models (Area Under the Receiver Operation Characteristics Curve [AUC] > 0.9) in predicting the poplar distribution, with RF achieving the best performance (r = 0.83 and AUC = 0.965), followed by SVM (r = 0.72 and AUC = 0.959) and then GLM (r = 0.65 and AUC = 0.937). In a geographical perspective, all models show a similar pattern, with the highest concentrations being in the south-western parts of the Western Cape, the Southern Cape on the Garden Route, the central-eastern Free State, Mpumalanga, and the southern parts of Limpopo. The evaluation of the relative importance of the bioclimates used showed that the warmest and driest quarter’s precipitation and annual precipitation significantly contribute to the poplar population. These results demonstrate the power of machine learning and regression models for predicting suitable habitats and extracting valuable environmental-climatic knowledge for monitoring and managing invasive tree species such as poplars.
Conservation implications: Poplars are among the most aggressive invasive plant species in South Africa. The results of this study are expected to help conservation authorities understand the current climatic factors affecting the species distribution, as well as potential sites.
Keywords: species distribution model; poplars; random forest; support vector machine; generalized linear model; bioclimates; alien invasive species.
Introduction
Species distribution modelling (SDM) has emerged as a powerful tool in ecological research (Peterson et al. 2011), greatly facilitating the understanding of geo-ecology and predicting the potentially suitable environment for species (Stewart et al. 2022). In principle, SDMs link defined species locations to predictor variables to evaluate patterns of species occurrence and habitat suitability (Elith, Kearney & Phillips 2010; Guisan, Thuiller & Zimmermann 2017; Wolmarans, Robertson & Van Rensburg 2010). The SDMs may even be used to predict species survival in response to future environmental shifts (Peterson et al. 2011). While SDMs often integrates’ climatic variables such as temperature and precipitation (Zhang et al. 2020), they also include other abiotic variables such as soil, land use, land cover and topography (Buri et al. 2017; Dubuis et al. 2013). Because of their fine spatial resolution for better fluctuations, the latter variables are included when the study aims to create SDM at local scale. Given their unique modelling ability, SDMs have become a popular strategy and a most widely used tool for characterising species distribution and predicting habitat suitability (Araújo et al. 2019).
Advances in computing resources and machine learning algorithms, as well as the availability of fine-scale remote sensing products and climate data are an important step forward in the use of SDMs for various applications (Guisan et al. 2017; Kass et al. 2021; Thuiller et al. 2009). These developments offer the opportunity to implement SDMs with great effect because the resolution at which they are computed greatly influence their overall performance (Lee-Yaw et al. 2022). Besides, the growing utility of SDMs lies in their relatively simple application with publicly available software packages, as well as low data requirements (Zurell 2019) and guidelines (Guisan et al. 2017).
Several studies have shown the high model performance in SDMs applications. In this regard, Zhang et al. (2020) predicted the possible distribution of Anredera cordifolia using Random Forest (RF) and also identified the temperature as the most important factor influencing the predictive performance of this species. Yudaputra, Pujiastuti and Cropper (2019) predicted the potential current distribution of Guettarda speciosa in Indonesia using the Maximum Entropy (MaxEnt), Support Vector Machine (SVM), RF, Generalized Linear Model (GLM), Domain, and Bioclim. Their study showed that MaxEnt outperformed other competitors with the highest area under the receiver operation characteristics curve (AUC) value of 0.89. Kaky et al. (2020) evaluated the performance of ensemble and MaxEnt modelling approaches in predicting the potential spread of Egyptian medicinal plants, where they found RF and MaxEnt performing better than SVM and Classification and Regression Tree (CART) in predicting the potential distribution of target species. However, the low performance of some models does not make them weak modelling approaches. Some models will perform poorly if all competency requirements are not met. For example, SVM sometimes performs better than MaxEnt and RF, possibly because the data structure is conducive to optimal performance. Therefore, ensuring that the model requirements are fully met before application and evaluation is essential. In general, studies have demonstrated high potential of machine learning and regression techniques for predicting species distributions in landscapes and ranking explanatory factors affecting distribution trends (Ahmed, Atzberger & Zewdie 2021; Shabani, Kumar & Ahmadi 2016). While these have been widely and successfully used to quantify the dispersal of many (native and non-native) floral species, they have not been applied in the study of Poplars in the Global South regions.
In South Africa, Populus alba and Populus canescens are classified as a Category 2 weed under the National Environmental Management and Biodiversity Act (NEMBA) (No. 10 of 2004) and Conservation of Agricultural Resources Act (CARA) (No. 43 of 1983) of South Africa’s Invasive Species Legislation (Henderson 2007). This means that poplars (Category 2) plants may not occur on land or inland water surfaces other than a demarcated area or a biological control reserve (Roy, Pauchard & Stoett 2023). For example, in most conservation areas under South African National Parks (SANParks), they are classified as a species of very high concern, encroaching on ecologically sensitive landscapes such as riparian zones; they are therefore cleared under continuous surveillance (SANParks 2020). Essentially, poplar species consumes a lot of water in streams, thereby worsening the conditions for aquatic species in the streams (Théroux Rancourt, Éthier & Pepin 2015). Like any woody species, poplar competes with native species for resources, with a better chance of consuming more and more because of its growth structure and crown, which intercepts rain and sunlight that would otherwise be used by native species (Kumschick et al. 2020; Zhang et al. 2021). For these reasons, it is important to understand their spatial distribution and climatic drivers so that they can be appropriately monitored and managed in the country. This study was conducted at national scale because at smaller spatial scale the bioclimatic variables do not give better variations (Fournier et al. 2017).
To achieve the aim of this study, the performance of GLM, RF and SVM models in predicting the existence and distribution of poplar trees in South Africa was tested and compared. The predictive power of each model under current different climatic conditions and evaluation of the most influential climatic parameters for Poplar distribution in the country was examined. The results of this study could provide valuable insights to support alien invasive species management actions and decision-making processes.
Research methods and design
Study area
This study was conducted in South Africa, the southernmost country on the African continent (Figure 1). The country lies between the 22–35° S and the 16–33° E (Van Wilgen et al. 2020). The country’s interior consists of a large, almost flat plateau with an altitude of about 1000 m in most places above the sea level (King 1942). South Africa has a temperate climate with warm and humid summers and cold and dry winters (Blamey et al. 2017). The country’s annual average rainfall is 464 mm, with the Western Cape receiving winter rainfall (June to August) and the rest of the country receiving summer rainfall (December to February) (Roffe, Fitchett & Curtis 2019). South Africa consists of nine vegetation biomes or units: Forests, Savannah, Fynbos, Grassland, Karoo, Desert, Nama-Karoo, Succulent Karoo, Indian Ocean Coastal Belts and Albany Thicket (Dayaram et al. 2019; Mucina & Rutherford 2006). Large impact craters, orogenic belts, granite belts and cratons are only a few examples of South Africa’s extremely diverse geology (Du Toit 1940). South Africa’s topography is made up of the craggy mountains of the Cape Fold Belt and the great escarpment, which encircle the country to the west, south and southeast. There is a narrow coastal plain strip beyond this (Haughton 1969).
 |
FIGURE 1: Depicts the distribution of different Populus species in South Africa. |
|
Data collection
To quantitatively predict poplar (Populus nigra, Populus simonii, Populus fremontii, Populus deltoides, P. canescens, Populus canadensis and P. alba) distribution in South Africa, spatially referenced poplar occurrence sample points were obtained from the Global Biodiversity Information Facility (GBIF) (https://www.gbif.org/ accessed on 12 June 2023 and updated on 20 December 2023). After filtering the data by South African boarder and removing duplicates, 467 poplar occurrence points were finally obtained and eligible for analysis. The species breakdown in terms of their locations is shown in Table 1. About 600 absence points were generated using the random point function in R environment.
TABLE 1: Poplar species gathered from global biodiversity information facility, including localities and counts. |
This study also attempted to identify the South African protected areas most likely to be affected by poplars by superimposing the best-modelled poplar distribution results on the protected area shapefiles and extracted protected areas where poplar occur using intersection function in Quantum Geographic Information System (QGIS). Only mostly affected protected areas are reported; however, the wider distribution is provided.
Environmental predictors
The Bioclim algorithm was used in this study; this is a commonly used climate-envelope-model (Booth et al. 2014) integrated into the R environment using a raster package, for environmental prediction from the Worldclim database (Table 2). Soil, land-use cover and topography have been documented as important environmental variables for plant species distribution (Huang et al. 2021; Krauss et al. 2008). However, in this study, the focus was only on the current climate variables affecting the poplar species’ range distribution in South Africa.
TABLE 2: Bioclimatic variables and their descriptions. |
All Bioclim variables were spatially scaled to a 1 km grid and cropped to the extent of the South African border. Temperature Bioclim in the Worldclim database is usually multiplied by 10 for storage purposes to reduce size. Temperature units were converted to degrees Celsius.
Modelling methods
This study was conducted using all available poplar species data as indicated in section ‘Distribution of poplar in South Africa’. Some species (e.g. Populus fremontii) had insufficient occurrence data and were unable to meet the modelling requirements for separate analyses. The commonly applied models for species distribution that include the GLMs (Melo-Merino, Reyes-Bonilla & Lira-Noriega 2020), RFs (Breiman 2001) and SVM (Vapnik 1998) were used in the R statistical environment. Stepwise logistic regression was used to select the best model combination. This process was set to repeat 10 times in a row to ensure that robust modelling output is achieved. The predictors from the model that yielded the lowest akaike information criterion (AIC) score were selected for modelling. This process is important as it allows for proper fitting of the model, which is a model that does not possess multicollinearity and data redundancy.
For all models, the same initial set of inputs was included, that is, poplar occurrence and absence points as response variable and 13 selected Bioclims as environmental predictors. Species presence was given value 1 and absence was given value 0 for modelling. Distribution data were divided into two parts, 75% for training and 25% for testing. The GLM was implemented using the ‘GLM’ function, link function and error distribution are given with the family arguments. Random Forests was implemented with ‘randomForest’ and the SVMs in R using the ‘ksvm’ in package ‘kernlab’ because it contains many different SVM formulations and ‘kernals’ and provides useful options and functions such as a method for plotting. Next, the RF was used to evaluate the most important variables (Genuer, Poggi & Tuleau-Malot 2010) in determining the occurrence and distribution of poplar in the South African landscape. All the models were set to repeat 10 times to ensure that our modelling results are consistent and accurate. The accuracy of all models was assessed by the area under the curve (AUC).
Ethical considerations
This article followed all ethical standards for research without direct contact with human or animal subjects.
Results
Model performance and validation
The geographic distribution of poplar trees (P. alba, P. canadensis, P. canescens, Populus deltoides, P. fremontii, Populus nigra and Populus simonii) were modelled in South Africa using GLM, RFs and SVM models, and their performance is shown in Table 3. The results show excellent performance for all models, with RF achieving the highest correlation of 0.83 and 0.965 AUC. This was followed by SVM and then GLM with correlation and AUC of 0.72 and 0.959, and 0.65 and 0.937, respectively (Table 3). A key feature of these results is the superior performance of machine learning methods than a regression model. In Figure 2, the comparison of the model accuracies with the receiver operator characteristics (ROC) curve is shown. Here, ROC shows the proportion of the true positive (presence) rate and the false positive (absence) rate for poplars. Higher RF AUC values indicate the ability to identify the presence and absence of poplar samples (Figure 2a), followed by SVM (Figure 2b) and then GLM (Figure 2c).
TABLE 3: A summary of model performance. |
 |
FIGURE 2: Receiver operator characteristics curve for (a) random forests, (b) support vector machine, and (c) generalised linear model. |
|
Distribution of poplar in South Africa
In this section, the results of the modelled poplar distribution are presented for South Africa using RF, SVM and GLM, and the results are shown in Figure 3. All models showed a similar pattern of poplar distribution, with higher concentration in the south-western part of the Western Cape, the southern Cape on the Garden Route, the central-eastern Free State, and the north-eastern part of the country in Mpumalanga, even in Limpopo (Figure 3a–c). However, RF had a better prediction performance than its counterparts (see Figure 2).
 |
FIGURE 3: Modelled distribution of poplars in South Africa using (a) random forests, (b) support vector machine, and (c) generalised linear model. |
|
Populus nigra, P. alba, and P. canescens appear to have suitable habitats throughout the rest of the country, except for the extremely hot and arid northern parts of the Northern Cape (Figure 4a). This conclusion was reached after overlaying all poplar species points on the results of a RFs modelling application. Many provinces have populations of P. deltoides; however, it has not been recorded in provinces such as the North West and the Free State. Nevertheless, there are just a few records of P. simonii (Western Cape), P. fremontii (KwaZulu-Natal), and P. canadensis (Free State and Mpumalanga) in South Africa (see Figure 4a). Overall, poplar species appear to be highly distributed in temperate regions with adequate rainfall patterns and less in arid deserted areas (see Figure 4b and Appendix 3). This is not surprising because the majority of poplar species require an inordinate amount of water to flourish and survive.
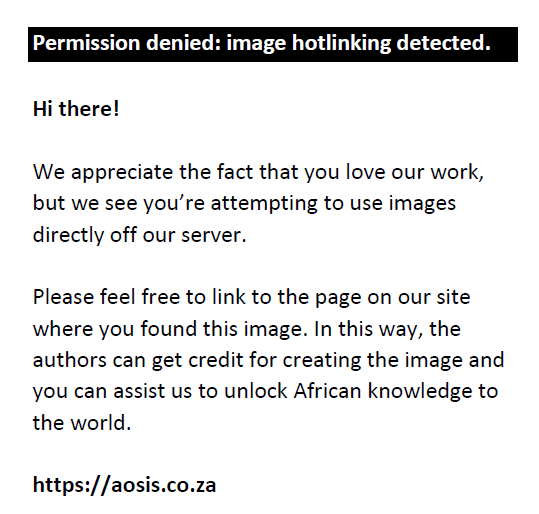 |
FIGURE 4: (a) Random forests prediction map and (b) a June 2022 to June 2023 rainfall map with poplar points overlayed on both. |
|
In assessing the occurrence status of poplars in South African protected areas, this study found that poplars are common in few protected areas, where conditions are more favourable. These include Golden Gate Highlands National Park, Table Mountain National Park, Cape Peninsula Nature Reserve, Tweefontein Reserve and the Platberg Private Nature Reserve to name but a few (see Appendix 1 and Appendix 2).
Of the 13 predictors used, Bio 4 (temperature seasonality), Bio 10 (mean temperature of the warmest quarter), Bio 11 (mean temperature of the coldest quarter), Bio 12 (annual precipitation), Bio 13 (precipitation of wettest month), Bio 14 (precipitation of the driest month), Bio 17 (precipitation of the driest quarter) and B 18 (precipitation of the warmest quarter) were found to be significant in determining the occurrence status of poplars (Table 4). The results seem to indicate that seasonal variation in temperatures and average temperatures of the warmest and coldest quarter are potential influencers of poplar distribution as far as temperature is concerned. From rainfall perspective, precipitation experienced annually, during the wettest month, driest month, driest quarter and warmest quarter are all integral in contributing towards the existence and invasion of poplar species in South Africa. Overall, the results suggest that regions that receive sufficient rainfall in all phases of the year and experience optimum temperatures during warm and coldest months are likely to host poplar occurrences.
TABLE 4: Evaluation of environmental factors for predicting the distributions of poplars. |
The study ranked the contribution of all 13 environmental variables in determining poplar occurrence and distribution and found the warmest and driest quarter precipitation, and annual precipitation to be the top three contributing factors (refer to Figure 5). The findings highlight the significance of wet conditions and water availability for poplar presence in South Africa.
 |
FIGURE 5: The importance of bioclimatic variables measured using random forests (a) percentage IncMSE and (b) IncNodePurity. |
|
Discussion
This study provided a successful prediction of poplar species distribution in South African landscapes and allowed the: (1) understanding of the geographic distribution and pattern of poplars in the country, (2) identification of environmental conditions that most affect poplar occurrences and dispersion, and (3) testing of the superior performance of Machine Learning models (RF and SVM), for modelling species distribution over a regression model.
The results showed that poplars are mainly found in regions of warm temperature and high rainfall in South Africa, including the south-western parts of the Western Cape and Northern Cape, the southern Cape on the Garden Route, the central-eastern Free State, western parts of KwaZulu-Natal, Gauteng, eastern parts of Northwest, the north-eastern part of the country in Mpumalanga and Limpopo. These also include protected areas found in the aforementioned regions, such as Golden Gate Highlands National Park (Daemane, Van Wyk & Moteetee 2010), Table Mountain National Park, Cape Peninsula Nature Reserve, Tweefontein Reserve, and Platberg Private Nature Reserve, to name but a few. The findings also seem to suggest that species such as P. nigra, P. alba, P. canescens, and P. deltoides are mostly found throughout the country, in the wetter and warmer provinces. Their ability to thrive in semi-drier to wetter conditions may cause this adaptation (Caudullo & De Rigo 2016; De Rigo et al. 2016). The majority develop themselves organically and thrive when enough water and sunlight are available. For windbreaking and decorative purposes, poplar species including P. simonii, P. fremontii, and P. canadensis are planted in homes and streets and are carefully tended to with frequent irrigation. Overall, the results are consistent with Ntshidi et al. (2018) and Mtengwana et al. (2021), because, from a geographic perspective, invasive species predominate in the wetter parts of the country, which are important water sources for the country’s major rivers. Poplar needs enough water and warmer temperatures for better growth and survival (Fischer et al. 2018; Kalcsits, Silim & Tanino 2009). This justifies their occurrence in riparian zones, and warmer and wetter parts of the country (Sperandio et al. 2022; Xi et al. 2021). While its invasiveness has not been extensively studied, like any other invasive species, it competes with native important species for resources, and at most outcompete them (Poudel et al. 2019; Zhang et al. 2021). Therefore, the results of this study are important as they enable the understanding of the patterns of their spread across the country and selected protected areas to know what interventions to put in place to monitor and manage them. In protected areas under the SANParks, poplar is categorised as species of special concern, which requires constant monitoring and management (SANParks 2020). However, knowledge about what constitutes to its spread is not yet available. Therefore, it is hoped that this gap will be filled as an extension of this study.
It is well known that the geospatial distribution of plants is largely influenced by an interplay of multiple mechanisms, including climate and other environmental characteristics (Chen et al. 2014). Although some of these parameters are not part of this modelling approach, they are likely to help determine poplar distribution. To some extent, the variations in topography and land cover are related to climatic variations (Huang et al. 2021; Krauss et al. 2008); hence, it is preferred to focus solely on the bioclimatic variables. Therefore, it is assumed that the observed distribution of the poplars depends solely on the current climate. For this reason, the RF was used to evaluate the most influential climatic variables. In doing so, this study found that warmest and driest quarter’s precipitation and annual precipitation are the most important variables in the prediction of poplar trees. In fact, warmer and humid conditions are favourable for poplars, while cold and dry conditions appear to be unsuitable. Likewise, Yudaputra, Robiansyah and Rinandio (2019) using RF, examined the variables essential to the dispersal of Eusideroxylon zwageri in Indonesia and found that precipitation in the coldest months, precipitation seasonality, and isothermality are the most important variables affecting the dispersal of the target species. This information is important for predicting the habitat suitability of landscapes to allow for the existence of species and thus their future spread (Burns, Clemann & White 2020; Zhang et al. 2019).
The results of this study are consistent with previous studies in which machine learning methods (RF and SVM) outperformed a regression model (GLM) (Ahmed et al. 2021). The superior performance of RF was also confirmed by Jensen et al. (2020) in their prediction of an invasive Kudzu vine in the USA using Sentinel-2. Random Forest uses only a random subset of the predictor variables for each subset as each tree grows. This creates decorrelated trees and reduces the variance of the final model (Hastie, Tibshirani & Friedman 2009). Additionally, RF avoids overfitting, although they can have quite a complex response, making RF the best model for modelling species distribution. As the second-best model, SVM works by constructing a series of hyperplanes to separate data points based on their class (Ahmed et al. 2021; Kass et al. 2021). Performance is often poor when the number of background samples significantly exceeds the occurrence records. This is largely due to class overlap (García-Roselló et al. 2019). This issue was combated by applying weights, which significantly increases the cost of misclassifying presence points (Duan et al. 2014). Support Vector Machine has been acknowledged for its performance in SDMs in many studies. For example, Bedia, Busqué and Gutiérrez (2011) used SVM and other models to predict the distribution of plant species in an alpine rangeland in northern Spain. Hailu et al. (2017) reported that SVM performed well together with MaxEnt in assessing the spatial distribution of Coffea arabica in the Ethiopian highlands. It is said that SVM essentially performs better than RF, especially when the number of observed occurrences is small, since it can be trained with few meaningful pixels (Pouteau et al. 2012). Generalized linear model is the least performing model in this study and is commonly used to determine the distribution pattern of species using the iterative weighted linear regression technique to obtain the estimated maximum likelihood of the parameters (Fukuda et al. 2013; Godsoe 2014). Its relative performance to RF and SVM is consistent with other similar studies. However, in some applications, the GLM has been found to perform better than the Boosted Regression Tree in predicting the distribution of eight different species in Australia (Shabani et al. 2016). Overall, all three models performed well as they achieved an AUC > 0.9, confirming their value to model species distributions, especially machine learning-based techniques.
Conclusions
This study predicted poplar distribution in South Africa using machine learning (RF and SVM) and regression (GLM) models. The results showed that poplars are mostly distributed in the warmer regions that receive the above average rainfall of the country, from the southwest of the Western Cape and Northern Cape, the central Free State, western parts of KwaZulu-Natal, eastern parts of North West, Mpumalanga, Gauteng, to the southern parts of Limpopo. This distribution appears to affect several protected areas from a conservation perspective, including the Golden Gate Highlands National Park, Table Mountain National Park, Cape Peninsula Nature Reserve, Tweefontein Reserve, and Platberg Private Nature Reserve to name but a few. The results of this study showed that machine learning methods (RF, 0.965 and SVM, 0.959) outperformed GLM (0.937) in predicting the occurrence and distribution of poplar trees under the current climatic conditions, with RF performing the best. Based on RF, the warmest and driest quarter’s precipitation and annual precipitation were the most influential climatic parameters for poplar distribution in South Africa. With continued evolution of SDMs, more poplar distribution data and availability are expected to further improve the understanding of species distribution and environmental variables that influence predictive performance.
Acknowledgements
Competing interests
The authors declare that they have no financial or personal relationship(s) that may have inappropriately influenced them in writing this article.
Authors’ contributions
Mr M.M. was involved in conceptualisation, methodology, formal analysis, investigation, writing- original draft, visualisation, project administration, software, validation, data curation, writing-review and editing and sourcing funds for the project. Dr S.X. was responsible for conceptualisation, writing-original draft, visualisation, software, validation, data curation, resources, writing-review and editing and supervision. Prof M.G. was involved in conceptualisation, writing-original draft, writing-review and editing and supervision.
Funding information
The study was funded by South African National Parks, Scientific Service, Kimberly.
Data availability
The data used to support the findings of this study are available from corresponding author upon request.
Disclaimer
The views and opinions expressed in this article are those of the authors and are the product of professional research. It does not necessarily reflect the official policy or position of any affiliated institution, funder, agency, or that of the publisher. The authors are responsible for this article’s results, findings, and content.
References
Ahmed, N., Atzberger, C. & Zewdie, W., 2021, ‘Species distribution modelling performance and its implication for Sentinel-2-based prediction of invasive Prosopis juliflora in lower Awash River basin, Ethiopia’, Ecological Processes 10(1), 1–16. https://doi.org/10.1186/s13717-021-00285-6
Araújo, M.B., Anderson, R.P., Márcia Barbosa, A., Beale, C.M., Dormann, C.F., Early, R. et al., 2019, ‘Standards for distribution models in biodiversity assessments’, Science Advances 5(1), 4858. https://doi.org/10.1126/sciadv.aat4858
Beck, H.E., Zimmermann, N.E., McVicar, T.R., Vergopolan, N., Berg, A. & Wood, E.F., 2018, ‘Present and future Köppen-Geiger climate classification maps at 1-km resolution’, Scientific Data 5(1), 1–12.
Bedia, J., Busqué, J. & Gutiérrez, J.M., 2011, ‘Predicting plant species distribution across an alpine rangeland in northern Spain. A comparison of probabilistic methods’, Applied Vegetation Science 14(3), 415–432. https://doi.org/10.1111/j.1654-109X.2011.01128.x
Blamey, R.C., Middleton, C., Lennard, C. & Reason, C.J.C., 2017, ‘A climatology of potential severe convective environments across South Africa’, Climate Dynamics 49, 2161–2178. https://doi.org/10.1007/s00382-016-3434-7
Booth, T.H., Nix, H.A., Busby, J.R. & Hutchinson, M.F., 2014, ‘BIOCLIM: The first species distribution modelling package, its early applications and relevance to most current MAXENT studies’, Diversity and Distributions 20, 1–9. https://doi.org/10.1111/ddi.12144
Breiman, L., 2001, ‘Random forests’, Machine Learning 45, 5–32. https://doi.org/10.1023/A:1010933404324
Buri, A., Cianfrani, C., Pinto-Figueroa, E., Yashiro, E., Spangenberg, J.E., Adatte, T. et al., 2017, ‘Soil factors improve predictions of plant species distribution in a mountain environment’, Progress in Physical Geography 41(6), 703–722. https://doi.org/10.1177/0309133317738162
Burns, P.A., Clemann, N. & White, M., 2020, ‘Testing the utility of species distribution modelling using random forests for a species in decline’, Austral Ecology 45(6), 706–716. https://doi.org/10.1111/aec.12884
Caudullo, G. & De Rigo, D., 2016, ‘Populus alba in Europe: Distribution, habitat, usage and threats’, in J. San-Miguel-Ayanz, D. De Rigo, G. Caudullo, T. Houston Durrant & A. Mauri (eds.), European atlas of forest tree species, pp. 134–135, Publications Office of the EU, Luxembourg.
Chen, Y., Yang, X., Yang, Q., Li, D., Long, W. & Luo, W., 2014, ‘Factors affecting the distribution pattern of wild plants with extremely small populations in Hainan Island, China’, PLoS One 9, e97751. https://doi.org/10.1371/journal.pone.0097751
Daemane, M.E., Van Wyk, B.E. & Moteetee, A., 2010, ‘Checklist of ferns and seed plants of the Golden Gate Highlands National Park, South Africa’, Bothalia 40(2), 205–218. https://doi.org/10.4102/abc.v40i2.222
Dayaram, A., Harris, L.R., Grobler, B.A., Van Der Merwe, S., Rebelo, A.G., Ward Powrie, L. et al., 2019, ‘Vegetation map of South Africa, Lesotho and Swaziland 2018: A description of changes since 2006’, Bothalia-African Biodiversity & Conservation 49(1), 1–11. https://doi.org/10.4102/abc.v49i1.2452
De Rigo, D., Enescu, C.M., Houston Durrant, T. & Caudullo, G., 2016, ‘Populus nigra in Europe: Distribution, habitat, usage and threats’, in J. San-Miguel-Ayanz, D. De Rigo, G. Caudullo, T.H. Durrant & A. Mauri (eds) European atlas of forest tree species, pp. 136–137.
Du Toit, A.L., 1940, ‘The geology of South Africa’, The Geographical Journal 95, 389. https://doi.org/10.2307/1787484
Duan, R.Y., Kong, X.Q., Huang, M.Y., Fan, W.Y. & Wang, Z.G., 2014, ‘The predictive performance and stability of six species distribution models’, PLoS One 9(11), e112764. https://doi.org/10.1371/journal.pone.0112764
Dubuis, A., Giovanettina, S., Pellissier, L., Pottier, J., Vittoz, P. & Guisan, A., 2013, ‘Improving the prediction of plant species distribution and community composition by adding edaphic to topo-climatic variables’, Journal of Vegetation Science 24(4), 593–606. https://doi.org/10.1111/jvs.12002
Elith, J., Kearney, M. & Phillips, S., 2010, ‘The art of modelling range-shifting species’, Methods in Ecology and Evolution 1(4), 330–342. https://doi.org/10.1111/j.2041-210X.2010.00036.x
Fischer, M., Zenone, T., Trnka, M., Orság, M., Montagnani, L., Ward, E.J. et al., 2018, ‘Water requirements of short rotation poplar coppice: Experimental and modelling analyses across Europe’, Agricultural and Forest Meteorology 250, 343–360. https://doi.org/10.1016/j.agrformet.2017.12.079
Fournier, A., Barbet-Massin, M., Rome, Q. & Courchamp, F., 2017, ‘Predicting species distribution combining multi-scale drivers’, Global Ecology and Conservation 12, 215–226. https://doi.org/10.1016/j.gecco.2017.11.002
Fukuda, S., De Baets, B., Waegeman, W., Verwaeren, J. & Mouton, A.M., 2013, ‘Habitat prediction and knowledge extraction for spawning European grayling (Thymallus thymallus L.) using a broad range of species distribution models’, Environmental Modelling & Software 47, 1–6. https://doi.org/10.1016/j.envsoft.2013.04.005
García-Roselló, E., Guisande, C., González-Vilas, L., González-Dacosta, J., Heine, J., Pérez-Costas, E. et al., 2019, ‘A simple method to estimate the probable distribution of species’, Ecography 42(9), 1613–1622. https://doi.org/10.1111/ecog.04563
Genuer, R., Poggi, J.M. & Tuleau-Malot, C., 2010, ‘Variable selection using random forests’, Pattern Recognition Letters 31, 2225–2236.
Godsoe, W., 2014, ‘Inferring the similarity of species distributions using species’ distribution models’, Ecography 37(2), 130–136. https://doi.org/10.1111/j.1600-0587.2013.00403.x
Guisan, A., Thuiller, W. & Zimmermann, N.E., 2017, Habitat suitability and distribution models with applications in R, Cambridge University Press, Cambridge.
Hailu, B.T., Siljander, M., Maeda, E.E. & Pellikka, P., 2017, ‘Assessing spatial distribution of Coffea arabica L. in Ethiopia’s highlands using species distribution models and geospatial analysis methods’, Ecological Informatics 42, 79–89. https://doi.org/10.1016/j.ecoinf.2017.10.001
Hastie, T., Tibshirani, R. & Friedman, J., 2009, The elements of statistical learning: Data mining, inference, and prediction, 2nd edn., Springer Series in Statistics, Springer, New York, NY.
Haughton, S.H., 1969, Geological Society of South Africa, Johannesburg, South Africa.
Henderson, L., 2007, ‘Invasive, naturalized and casual alien plants in southern Africa: A summary based on the Southern African Plant Invaders Atlas (SAPIA)’, Bothalia 37(2), 215–248. https://doi.org/10.4102/abc.v37i2.322
Huang, E., Chen, Y., Fang, M., Zheng, Y. & Yu, S., 2021, ‘Environmental drivers of plant distributions at global and regional scales’, Global Ecology and Biogeography 30(3), 697–709. https://doi.org/10.1111/geb.13251
Jensen, T., Seerup Hass, F., Seam Akbar, M., Holm Petersen, P. & Jokar Arsanjani, J., 2020, ‘Employing machine learning for detection of invasive species using sentinel-2 and aviris data: The case of Kudzu in the United States’, Sustainability 12(9), 3544. https://doi.org/10.3390/su12093544
Kaky, E., Nolan, V., Alatawi, A. & Gilbert, F., 2020, ‘A comparison between Ensemble and MaxEnt species distribution modelling approaches for conservation: A case study with Egyptian medicinal plants’, Ecological Informatics 60, 101150. https://doi.org/10.1016/j.ecoinf.2020.101150
Kalcsits, L.A., Silim, S. & Tanino, K., 2009, ‘Warm temperature accelerates short photoperiod-induced growth cessation and dormancy induction in hybrid poplar (Populus× spp.)’, Trees 23, 971–979. https://doi.org/10.1007/s00468-009-0339-7
Kass, J.M., Meenan, S.I., Tinoco, N., Burneo, S.F. & Anderson, R.P., 2021, ‘Improving area of occupancy estimates for parapatric species using distribution models and support vector machines’, Ecological Applications 31(1), e02228. https://doi.org/10.1002/eap.2228
King, L.C., 1942, South African scenery. A textbook of geomorphology, Oliver and Boyd, London, UK.
Krauss, K.W., Lovelock, C.E., McKee, K.L., López-Hoffman, L., Ewe, S.M. & Sousa, W.P., 2008, ‘Environmental drivers in mangrove establishment and early development: A review’, Aquatic Botany 89(2), 105–127. https://doi.org/10.1016/j.aquabot.2007.12.014
Kumschick, S., Bacher, S., Bertolino, S., Blackburn, T.M., Evans, T., Roy, H.E. et al., 2020, ‘Appropriate uses of EICAT protocol, data and classifications’, NeoBiota 62, 193–212. https://doi.org/10.3897/neobiota.62.51574
Lee-Yaw, A.J., McCune, L.J., Pironon, S. & Sheth, N.S., 2022, ‘Species distribution models rarely predict the biology of real populations’, Ecography 2022(6), e05877. https://doi.org/10.1111/ecog.05877
Melo-Merino, S.M., Reyes-Bonilla, H. & Lira-Noriega, A., 2020, ‘Ecological niche models and species distribution models in marine environments: A literature review and spatial analysis of evidence’, Ecological Modelling 415, 108837. https://doi.org/10.1016/j.ecolmodel.2019.108837
Mtengwana, B., Dube, T., Mudereri, B.T. & Shoko, C., 2021, ‘Modeling the geographic spread and proliferation of invasive alien plants (IAPs) into new ecosystems using multi-source data and multiple predictive models in the Heuningnes catchment, South Africa’, GIScience & Remote Sensing 58(4), 483–500. https://doi.org/10.1080/15481603.2021.1903281
Mucina, L. & Rutherford, M.C., 2006, ‘The vegetation of South Africa, Lesotho and Swaziland’, Strelitzia 19, 1–30, viewed 23 February 2022, from http://ebooks.cambridge.org/ref/id/CBO9781107415324A009.
Ntshidi, Z., Gush, M.B., Dzikiti, S. & Le Maitre, D.C., 2018, ‘Characterising the water use and hydraulic properties of riparian tree invasions: A case study of Populus canescens in South Africa’, Water SA 44, 328–337. https://doi.org/10.4314/wsa.v44i2.18
Peterson, A.T., Soberon, J., Pearson, R.G., Anderson, R.P., Martinez-Meyer, E., Nakamura, M. et al., 2011, Ecological niches and geographic distributions, Princeton University Press, Princeton, NJ, USA.
Poudel, D.R., Chen, H.Y., KC, M., Ge, Z., Bown, H.E. & Ruan, H., 2019, ‘Understory vegetation dynamics across a poplar plantation chronosequence in reclaimed coastal saline soil’, Forests 10(9), 764. https://doi.org/10.3390/f10090764
Pouteau, R., Meyer, J.Y., Taputuarai, R. & Stoll, B., 2012, ‘Support vector machines to map rare and endangered native plants in Pacific islands forests’, Ecological Informatics 9, 37–46. https://doi.org/10.1016/j.ecoinf.2012.03.003
Roffe, S.J., Fitchett, J.M. & Curtis, C.J., 2019, ‘Classifying and mapping rainfall seasonality in South Africa: A review’, South African Geographical Journal = Suid-Afrikaanse Geografiese Tydskrif 101(2), 158–174. https://doi.org/10.1080/03736245.2019.1573151
Roy, H.E., Pauchard, A., Stoett, P., Renard Truong, T., Bacher, S., Galil, B.S. et al., 2023, ‘IPBES invasive alien species assessment: Summary for policymakers (Version 2)’, IPBES secretariat, Bonn, Germany, viewed n.d., from https://doi.org/10.5281/zenodo.8314303
SANParks, 2020, Golden gate highlands national park management plan, SANParks, Pretoria.
Shabani, F., Kumar, L. & Ahmadi, M., 2016, ‘A comparison of absolute performance of different correlative and mechanistic species distribution models in an independent area’, Ecology and Evolution 6(16), 5973–5986. https://doi.org/10.1002/ece3.2332
Sperandio, G., Pagano, M., Acampora, A., Civitarese, V., Cedrola, C., Mattei, P. et al., 2022, ‘Deficit irrigation for efficiency and water saving in poplar plantations’, Sustainability 14(21), 13991. https://doi.org/10.3390/su142113991
Stewart, S.B., Fedrigo, M., Kasel, S., Roxburgh, S.H., Choden, K., Tenzin, K. et al., 2022, ‘Predicting plant species distributions using climate-based model ensembles with corresponding measures of congruence and uncertainty’, Diversity and Distributions 28(5), 1105–1122. https://doi.org/10.1111/ddi.13515
Théroux Rancourt, G., Éthier, G. & Pepin, S., 2015, ‘Greater efficiency of water use in poplar clones having a delayed response of mesophyll conductance to drought’, Tree Physiology 35(2), 172–184. https://doi.org/10.1093/treephys/tpv006
Thuiller, W., Lafourcade, B., Engler, R. & Araújo, M.B., 2009, ‘BIOMOD – A platform for ensemble forecasting of species distributions’, Ecography 32, 369–373. https://doi.org/10.1111/j.1600-0587.2008.05742.x
Van Wilgen, B.W., Measey, J., Richardson, D.M., Wilson, J.R. & Zengeya, T.A., 2020, Biological invasions in South Africa, p. 975, Springer Nature, Knoxville, TN.
Vapnik, V., 1998, Statistical learning theory, Wiley, New York, NY.
Wolmarans, R., Robertson, M.P. & Van Rensburg, B.J., 2010, ‘Predicting invasive alien plant distributions: How geographical bias in occurrence records influences model performance’, Journal of Biogeography 37(9), 1797–1810. https://doi.org/10.1111/j.1365-2699.2010.02325.x
Xi, B., Clothier, B., Coleman, M., Duan, J., Hu, W., Li, D. et al., 2021, ‘Irrigation management in poplar (Populus spp.) plantations: A review’, Forest Ecology and Management 494, 119330. https://doi.org/10.1016/j.foreco.2021.119330
Yudaputra, A., Pujiastuti, I. & Cropper, W.P., 2019, ‘Comparing six different species distribution models with several subsets of environmental variables: Predicting the potential current distribution of Guettarda speciosa in Indonesia’, Biodiversitas Journal of Biological Diversity 20(8), 2321–2328. https://doi.org/10.13057/biodiv/d200830
Yudaputra, A., Robiansyah, I. & Sumeru, R.D., 2019, ‘The implementation of artifcial neural network and random forest in ecological research: Species distribution modelling with presence and absence dataset’, Research Center for Plant Conservation and Botanic Gardens, Indonesian Institute of Sciences, Jl. Ir. H. Juanda No. 13, Bogor, Indonesia.
Zhang, J., Qin, G., Zhai, Z., Zhou, S., Tang, L. & Tian, Y., 2021, ‘Diverse understory vegetation alleviates nitrogen competition with crop trees in poplar plantations’, Forests 12(6), 705. https://doi.org/10.3390/f12060705
Zhang, K., Zhang, Y., Jia, D. & Tao, J., 2020, ‘Species distribution modeling of Sassafras tzumu and implications for forest management’, Sustainability 12(10), 4132. https://doi.org/10.3390/su12104132
Zhang, L., Huettmann, F., Liu, S., Sun, P., Yu, Z., Zhang, X. et al., 2019, ‘Classification and regression with random forests as a standard method for presence-only data SDMs: A future conservation example using China tree species’, Ecological Informatics 52, 46–56. https://doi.org/10.1016/j.ecoinf.2019.05.003
Zurell, D., 2019, Introduction to species distribution modelling (SDM) in R, viewed 10 March 2023, from https://damariszurell.github.io/SDM-Intro/.
Appendix 1
 |
FIGURE 1-A1: Protected areas overlaid on the poplar habitat suitability map to represent protected areas best suited for poplar growth. |
|
Appendix 2
TABLE 1-A2: A list of protected areas where poplars occur. |
Appendix 3
 |
FIGURE 1-A3: South African Climatic Zones with poplar species overlayed. |
|
|